pkgs <- c("Biobase", "arrayQualityMetrics", "dplyr", "knitr", "mclust", "pQTLtools", "rgl", "scatterplot3d")
for (p in pkgs) if (length(grep(paste("^package:", p, "$", sep=""), search())) == 0) {
if (!requireNamespace(p)) warning(paste0("This vignette needs package `", p, "'; please install"))
}
invisible(suppressMessages(lapply(pkgs, require, character.only = TRUE)))
We start with Bioconductor/Biobase’s ExpressionSet example and finish with a real application.
dataDirectory <- system.file("extdata", package="Biobase")
exprsFile <- file.path(dataDirectory, "exprsData.txt")
exprs <- as.matrix(read.table(exprsFile, header=TRUE, sep="\t", row.names=1, as.is=TRUE))
pDataFile <- file.path(dataDirectory, "pData.txt")
pData <- read.table(pDataFile, row.names=1, header=TRUE, sep="\t")
all(rownames(pData)==colnames(exprs))
metadata <- data.frame(labelDescription=c("Patient gender",
"Case/control status",
"Tumor progress on XYZ scale"),
row.names=c("gender", "type", "score"))
phenoData <- Biobase::AnnotatedDataFrame(data=pData, varMetadata=metadata)
experimentData <- Biobase::MIAME(name="Pierre Fermat",
lab="Francis Galton Lab",
contact="pfermat@lab.not.exist",
title="Smoking-Cancer Experiment",
abstract="An example ExpressionSet",
url="www.lab.not.exist",
other=list(notes="Created from text files"))
exampleSet <- pQTLtools::make_ExpressionSet(exprs,phenoData,experimentData=experimentData,
annotation="hgu95av2")
data(sample.ExpressionSet, package="Biobase")
identical(exampleSet,sample.ExpressionSet)
1 data.frame
The great benefit is to use the object directly as a data.frame.
lm.result <- Biobase::esApply(exampleSet,1,function(x) lm(score~gender+x))
beta.x <- unlist(lapply(lapply(lm.result,coef),"[",3))
beta.x[1]
#> AFFX-MurIL2_at.x
#> -0.0001907472
lm(score~gender+AFFX.MurIL2_at,data=exampleSet)
#>
#> Call:
#> lm(formula = score ~ gender + AFFX.MurIL2_at, data = exampleSet)
#>
#> Coefficients:
#> (Intercept) genderMale AFFX.MurIL2_at
#> 0.5531725 0.0098932 -0.0001907
2 Composite plots
We wish to examine the distribution of each feature via histogram, scatter and boxplot.
One could resort to esApply()
for its simplicity as before
invisible(Biobase::esApply(exampleSet[1:2],1,function(x)
{par(mfrow=c(3,1));boxplot(x);hist(x);plot(x)}
))
but it is nicer to add feature name in the title.
par(mfrow=c(1,3))
f <- Biobase::featureNames(exampleSet[1:2])
invisible(sapply(f,function(x) {
d <- t(Biobase::exprs(exampleSet[x]))
fn <- Biobase::featureNames(exampleSet[x])
hist(d,main="",xlab=fn); plot(d, ylab=fn); boxplot(d,ylab=fn)
}
)
)
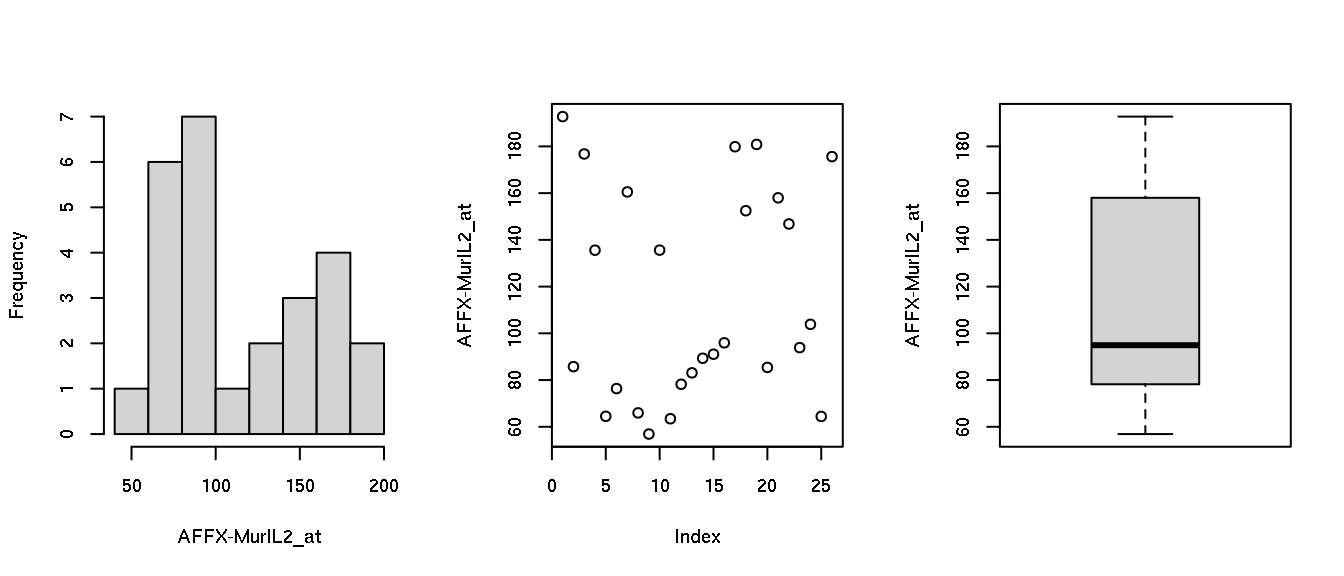
Figure 2.1: Histogram, scatter & boxplots
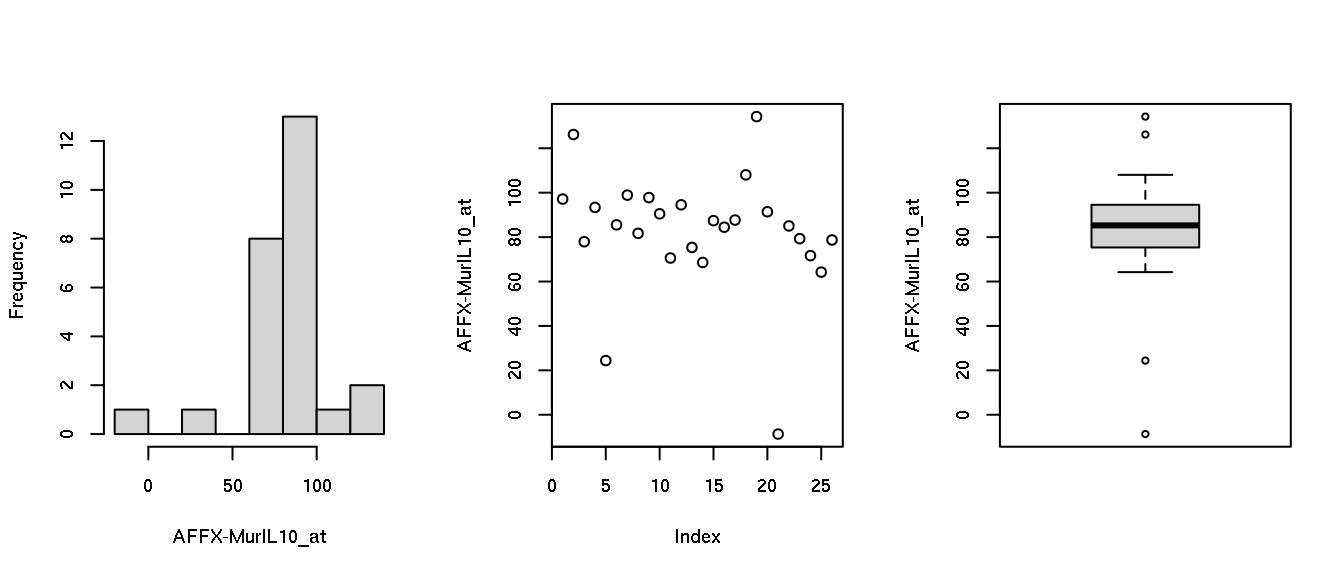
Figure 2.2: Histogram, scatter & boxplots
where the expression set is indexed using feature name(s).
4 Clustering
We employ model-based clustering absed on principal compoents to see potential groupings in the data,
pca <- prcomp(na.omit(t(Biobase::exprs(exampleSet))), rank=10, scale=TRUE)
pc1pc2pc3 <- with(pca,x)[,1:3]
mc <- mclust::Mclust(pc1pc2pc3,G=3)
with(mc, {
cols <- c("blue","red", "purple")
s3d <- scatterplot3d::scatterplot3d(with(pca,x[,c(2,1,3)]),
color=cols[classification],
pch=16,
type="h",
main="Plot of the PC1, PC2 and PC3")
s3d.coords <- s3d$xyz.convert(with(pca,x[,c(2,1,3)]))
text(s3d.coords$x,
s3d.coords$y,
cex = 1.2,
col = cols[classification],
labels = row.names(pc1pc2pc3),
pos = 4)
legend("right", legend=levels(as.factor(classification)), col=cols[classification], pch=16)
rgl::open3d(width = 500, height = 500)
rgl::plot3d(with(pca,x[,c(2,1,3)]),cex=1.2,col=cols[classification],size=5)
rgl::text3d(with(pca,x[,c(2,1,3)]),cex=1.2,col=cols[classification],texts=row.names(pc1pc2pc3))
htmlwidgets::saveWidget(rgl::rglwidget(), file = "mcpca3d.html")
})
#> Warning in par3d(userMatrix = structure(c(1, 0, 0, 0, 0, 0.342020143325668, :
#> parameter "width" cannot be set
#> Warning in par3d(userMatrix = structure(c(1, 0, 0, 0, 0, 0.342020143325668, :
#> parameter "height" cannot be set
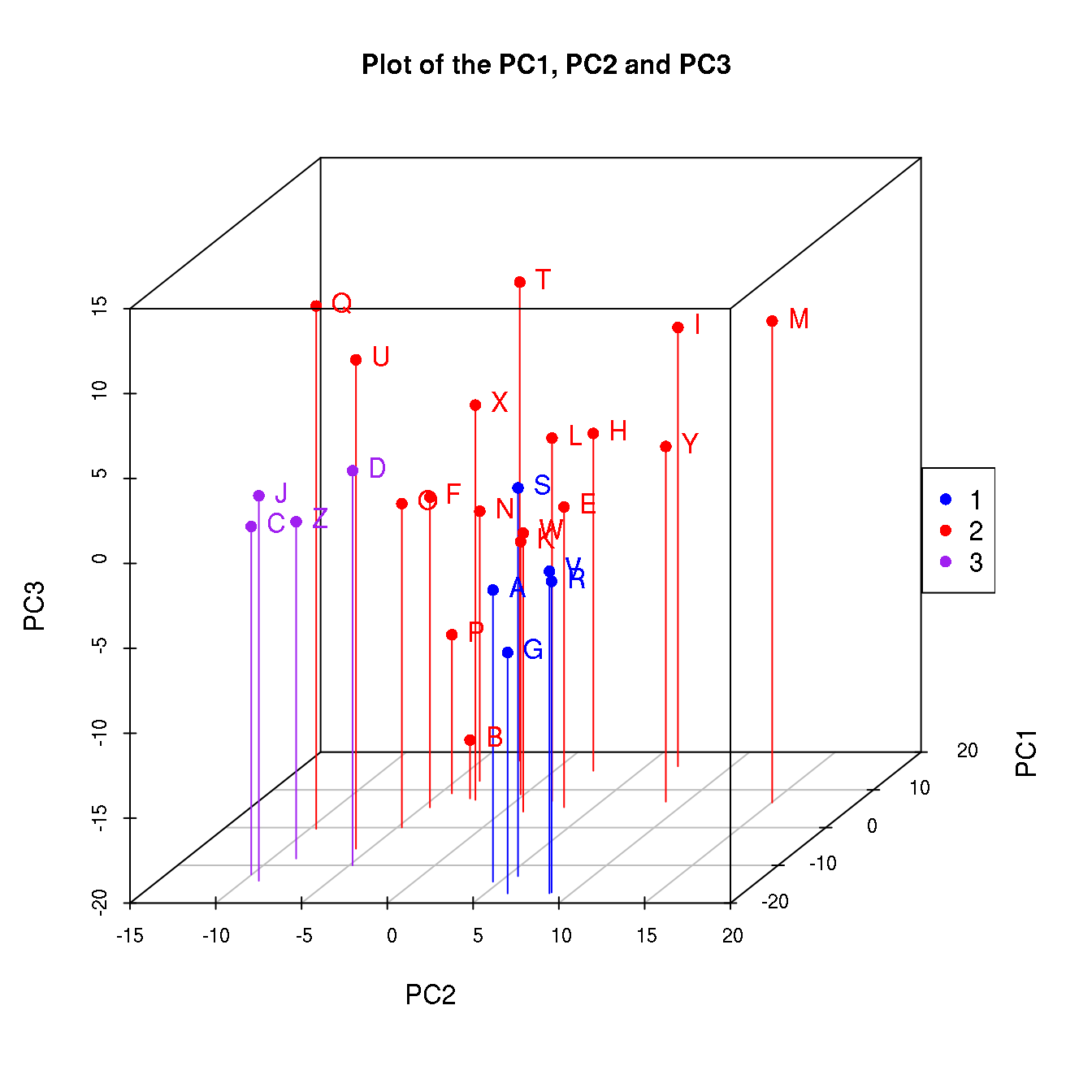
Figure 4.1: Three-group Clustering
An interactive version is also available,
5 Data transformation
Suppose we wish use log2 for those greater than zero but set those negative values to be missing.
6 Limit of detection (LOD)
We generate a lod.max
~ U[0,1] variable to experiment
Biobase::fData(exampleSet)
#> data frame with 0 columns and 500 rows
Biobase::fData(exampleSet)$lod.max <- apply(Biobase::exprs(exampleSet),1,quantile,runif(nrow(exampleSet)))
lod <- pQTLtools::get.prop.below.LLOD(exampleSet)
x <- dplyr::arrange(Biobase::fData(lod),desc(pc.belowLOD.new))
knitr::kable(head(lod))
AFFX.MurIL2_at | AFFX.MurIL10_at | AFFX.MurIL4_at | AFFX.MurFAS_at | AFFX.BioB.5_at | AFFX.BioB.M_at | gender | type | score | |
---|---|---|---|---|---|---|---|---|---|
A | 192.7420 | 97.13700 | 45.81920 | 22.54450 | 96.7875 | 89.0730 | Female | Control | 0.75 |
B | 85.7533 | 126.19600 | 8.83135 | 3.60093 | 30.4380 | 25.8461 | Male | Case | 0.40 |
C | 176.7570 | 77.92160 | 33.06320 | 14.68830 | 46.1271 | 57.2033 | Male | Control | 0.73 |
D | 135.5750 | 93.37130 | 28.70720 | 12.33970 | 70.9319 | 69.9766 | Male | Case | 0.42 |
E | 64.4939 | 24.39860 | 5.94492 | 36.86630 | 56.1744 | 49.5822 | Female | Case | 0.93 |
F | 76.3569 | 85.50880 | 28.29250 | 11.25680 | 42.6756 | 26.1262 | Male | Control | 0.22 |
G | 160.5050 | 98.90860 | 30.96940 | 23.00340 | 86.5156 | 75.0083 | Male | Case | 0.96 |
H | 65.9631 | 81.69320 | 14.79230 | 16.21340 | 30.7927 | 42.3352 | Male | Case | 0.79 |
I | 56.9039 | 97.80150 | 14.23990 | 12.03750 | 19.7183 | 41.1207 | Female | Case | 0.37 |
J | 135.6080 | 90.48380 | 34.48740 | 4.54978 | 46.3520 | 91.5307 | Male | Control | 0.63 |
K | 63.4432 | 70.57330 | 20.35210 | 8.51782 | 39.1326 | 39.9136 | Male | Case | 0.26 |
L | 78.2126 | 94.54180 | 14.15540 | 27.28520 | 41.7698 | 49.8397 | Female | Control | 0.36 |
M | 83.0943 | 75.34550 | 20.62510 | 10.16160 | 80.2197 | 63.4794 | Male | Case | 0.41 |
N | 89.3372 | 68.58270 | 15.92310 | 20.24880 | 36.4903 | 24.7007 | Male | Case | 0.80 |
O | 91.0615 | 87.40500 | 20.15790 | 15.78490 | 36.4021 | 47.4641 | Female | Case | 0.10 |
P | 95.9377 | 84.45810 | 27.81390 | 14.32760 | 35.3054 | 47.3578 | Female | Control | 0.41 |
Q | 179.8450 | 87.68060 | 32.79110 | 15.94880 | 58.6239 | 58.1331 | Female | Case | 0.16 |
R | 152.4670 | 108.03200 | 33.52920 | 14.67530 | 114.0620 | 104.1220 | Male | Control | 0.72 |
S | 180.8340 | 134.26300 | 19.81720 | -7.91911 | 93.4402 | 115.8310 | Male | Case | 0.17 |
T | 85.4146 | 91.40310 | 20.41900 | 12.88750 | 22.5168 | 58.1224 | Female | Case | 0.74 |
U | 157.9890 | -8.68811 | 26.87200 | 11.91860 | 48.6462 | 73.4221 | Male | Control | 0.35 |
V | 146.8000 | 85.02120 | 31.14880 | 12.83240 | 90.2215 | 64.6066 | Female | Control | 0.77 |
W | 93.8829 | 79.29980 | 22.34200 | 11.13900 | 42.0053 | 40.3068 | Male | Control | 0.27 |
X | 103.8550 | 71.65520 | 19.01350 | 7.55564 | 57.5738 | 41.8209 | Male | Control | 0.98 |
Y | 64.4340 | 64.23690 | 12.16860 | 19.98490 | 44.8216 | 46.1087 | Female | Case | 0.94 |
Z | 175.6150 | 78.70680 | 17.37800 | 8.96849 | 61.7044 | 49.4122 | Female | Case | 0.32 |
plot(x[,2], main="Random quantile cutoff", ylab="<lod%")
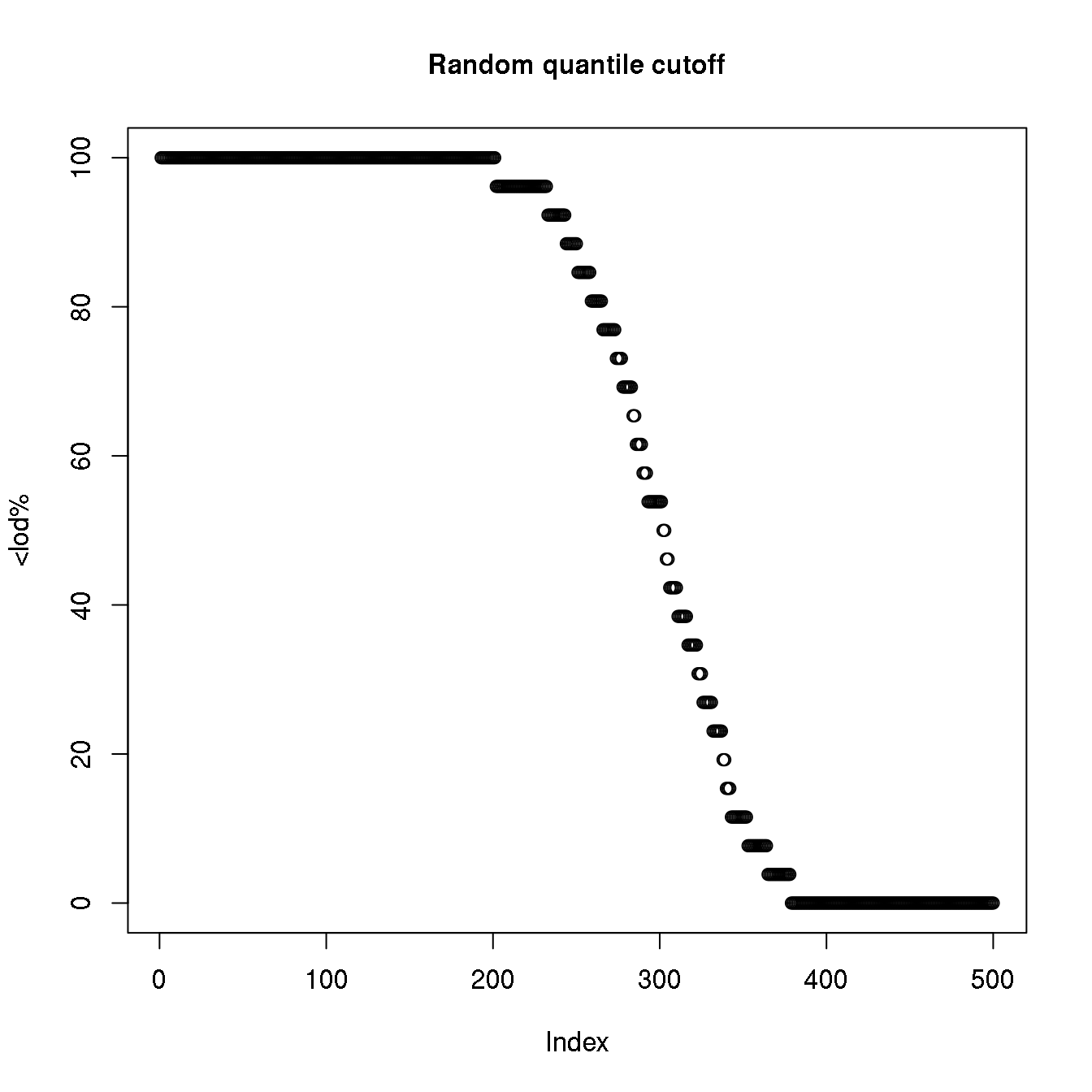
Figure 6.1: LOD based on a random cutoff
The quantity has been shown to have a big impact on protein abundance and therefore pQTL detection as is shown with a real example.
rm(list=ls())
dir <- "~/rds/post_qc_data/interval/phenotype/olink_proteomics/post-qc/"
eset <- readRDS(paste0(dir,"eset.inf1.flag.out.outlier.in.rds"))
x <- pQTLtools::get.prop.below.LLOD(eset)
annot <- Biobase::fData(x)
annot$MissDataProp <- as.numeric(gsub("\\%$", "", annot$MissDataProp))
plot(annot$MissDataProp, annot$pc.belowLOD.new, xlab="% <LLOD in Original",
ylab="% <LLOD in post QC dataset", pch=19)
INF <- Sys.getenv("INF")
np <- read.table(paste(INF, "work", "INF1.merge.nosig", sep="/"), header=FALSE,
col.names = c("prot", "uniprot"))
kable(np, caption="Proteins with no pQTL")
annot$pQTL <- rep(NA, nrow(annot))
no.pQTL.ind <- which(annot$uniprot.id %in% np$uniprot)
annot$pQTL[no.pQTL.ind] <- "red"
annot$pQTL[-no.pQTL.ind] <- "blue"
annot <- annot[order(annot$pc.belowLOD.new, decreasing = TRUE),]
annot <- annot[-grep("^BDNF$", annot$ID),]
saveRDS(annot,file=file.path("~","pQTLtools","tests","annot.RDS"))
annot <- readRDS(file.path(find.package("pQTLtools"),"tests","annot.RDS")) %>%
dplyr::left_join(pQTLdata::inf1[c("prot","target.short","alt_name")],by=c("ID"="prot")) %>%
dplyr::mutate(prot=if_else(is.na(alt_name),target.short,alt_name),order=1:n()) %>%
dplyr::arrange(desc(order))
xtick <- seq(1, nrow(annot))
attach(annot)
par(mar=c(10,5,1,1))
plot(100-pc.belowLOD.new,cex=2,pch=19,col=pQTL,xaxt="n",xlab="",ylab="",cex.axis=0.8)
text(66,16,"IL-17C",offset=0,pos=2,cex=1.5,font=2,srt=0)
arrows(67,16,71,16,lwd=2)
axis(1, at=xtick, labels=prot, lwd.tick=0.5, lwd=0, las=2, hadj=1, cex.axis=0.8)
mtext("% samples above LLOD",side=2,line=2.5,cex=1.2)
mtext("Ordered protein",side=1,line=6.5,cex=1.2,font=1)
legend(x=1,y=25,c("without pQTL","with pQTL"),box.lwd=0,cex=2,col=c("red","blue"),pch=19)
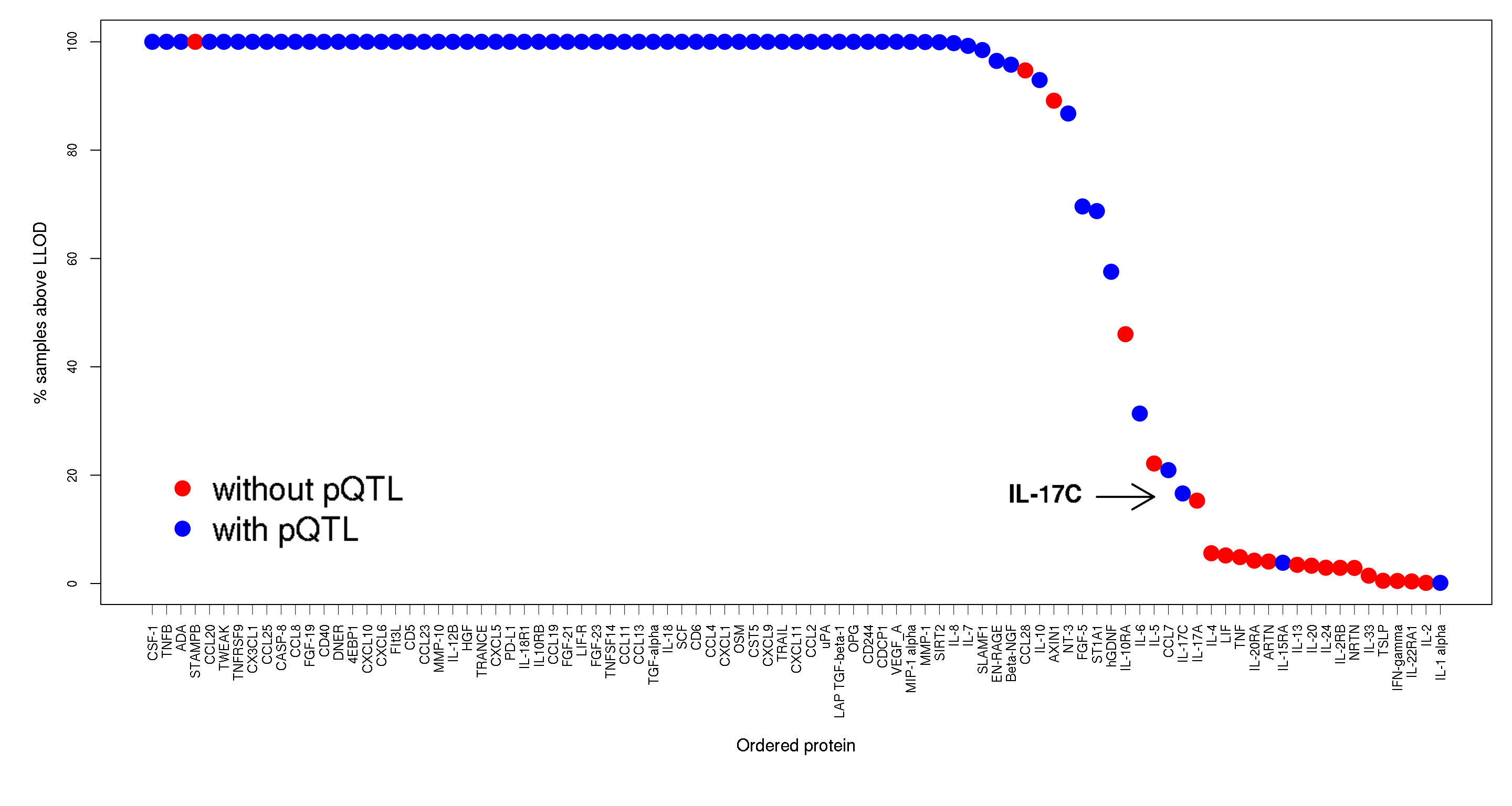
Figure 6.2: LOD in SCALLOP-INF/INTERVAL
ID | dichot | olink.id | uniprot.id | lod.max | MissDataProp | pc.belowLOD.new | pQTL | target.short | alt_name | prot | order |
---|---|---|---|---|---|---|---|---|---|---|---|
CSF.1 | FALSE | 196_CSF-1 | P09603 | 1.02 | 0.02 | 0.0000000 | blue | CSF-1 | NA | CSF-1 | 91 |
TNFB | FALSE | 195_TNFB | P01374 | 1.10 | 0.04 | 0.0000000 | blue | TNFB | NA | TNFB | 90 |
ADA | FALSE | 194_ADA | P00813 | 1.80 | 0.04 | 0.0000000 | blue | ADA | NA | ADA | 89 |
STAMPB | FALSE | 192_STAMPB | O95630 | 1.40 | 0.06 | 0.0000000 | red | STAMPB | NA | STAMPB | 88 |
CCL20 | FALSE | 190_CCL20 | P78556 | 1.42 | 0.02 | 0.0000000 | blue | CCL20 | NA | CCL20 | 87 |
TWEAK | FALSE | 189_TWEAK | O43508 | 1.78 | 0.02 | 0.0000000 | blue | TWEAK | NA | TWEAK | 86 |
TNFRSF9 | FALSE | 187_TNFRSF9 | Q07011 | 1.86 | 0.02 | 0.0000000 | blue | TNFRSF9 | NA | TNFRSF9 | 85 |
CX3CL1 | FALSE | 186_CX3CL1 | P78423 | 1.76 | 0.02 | 0.0000000 | blue | CX3CL1 | NA | CX3CL1 | 84 |
CCL25 | FALSE | 185_CCL25 | O15444 | 1.32 | 0.02 | 0.0000000 | blue | CCL25 | NA | CCL25 | 83 |
CASP.8 | FALSE | 184_CASP-8 | Q14790 | 1.38 | 0.04 | 0.0000000 | blue | CASP-8 | NA | CASP-8 | 82 |
MCP.2 | FALSE | 183_MCP-2 | P80075 | 2.10 | 0.02 | 0.0000000 | blue | MCP-2 | CCL8 | CCL8 | 81 |
FGF.19 | FALSE | 179_FGF-19 | O95750 | 1.25 | 0.02 | 0.0000000 | blue | FGF-19 | NA | FGF-19 | 80 |
CD40 | FALSE | 176_CD40 | P25942 | 1.91 | 0.04 | 0.0000000 | blue | CD40 | NA | CD40 | 79 |
DNER | FALSE | 174_DNER | Q8NFT8 | 1.63 | 0.02 | 0.0000000 | blue | DNER | NA | DNER | 78 |
4E.BP1 | FALSE | 170_4E-BP1 | Q13541 | 1.04 | 0.10 | 0.0000000 | blue | 4EBP1 | NA | 4EBP1 | 77 |
CXCL10 | FALSE | 169_CXCL10 | P02778 | 2.03 | 0.02 | 0.0000000 | blue | CXCL10 | NA | CXCL10 | 76 |
CXCL6 | FALSE | 168_CXCL6 | P80162 | 1.80 | 0.02 | 0.0000000 | blue | CXCL6 | CXCL6 | CXCL6 | 75 |
Flt3L | FALSE | 167_Flt3L | P49771 | 2.12 | 0.02 | 0.0000000 | blue | FIt3L | NA | FIt3L | 74 |
CD5 | FALSE | 165_CD5 | P06127 | 1.42 | 0.02 | 0.0000000 | blue | CD5 | NA | CD5 | 73 |
CCL23 | FALSE | 164_CCL23 | P55773 | 2.10 | 0.02 | 0.0000000 | blue | CCL23 | NA | CCL23 | 72 |
MMP.10 | FALSE | 161_MMP-10 | P09238 | 1.56 | 0.02 | 0.0000000 | blue | MMP-10 | NA | MMP-10 | 71 |
IL.12B | FALSE | 157_IL-12B | P29460 | 0.85 | 0.02 | 0.0000000 | blue | IL-12B | NA | IL-12B | 70 |
HGF | FALSE | 156_HGF | P14210 | 1.77 | 0.02 | 0.0000000 | blue | HGF | NA | HGF | 69 |
TRANCE | FALSE | 155_TRANCE | O14788 | 1.51 | 0.04 | 0.0000000 | blue | TRANCE | NA | TRANCE | 68 |
CXCL5 | FALSE | 154_CXCL5 | P42830 | 1.92 | 0.02 | 0.0000000 | blue | CXCL5 | NA | CXCL5 | 67 |
PD.L1 | FALSE | 152_PD-L1 | Q9NZQ7 | 2.14 | 0.04 | 0.0000000 | blue | PD-L1 | NA | PD-L1 | 66 |
IL.18R1 | FALSE | 151_IL-18R1 | Q13478 | 1.21 | 0.02 | 0.0000000 | blue | IL-18R1 | NA | IL-18R1 | 65 |
IL.10RB | FALSE | 149_IL-10RB | Q08334 | 1.72 | 0.02 | 0.0000000 | blue | IL10RB | NA | IL10RB | 64 |
CCL19 | FALSE | 145_CCL19 | Q99731 | 2.13 | 0.02 | 0.0000000 | blue | CCL19 | NA | CCL19 | 63 |
FGF.21 | FALSE | 144_FGF-21 | Q9NSA1 | 1.14 | 0.02 | 0.0000000 | blue | FGF-21 | NA | FGF-21 | 62 |
LIF.R | FALSE | 143_LIF-R | P42702 | 2.08 | 0.04 | 0.0000000 | blue | LIF-R | NA | LIF-R | 61 |
FGF.23 | FALSE | 139_FGF-23 | Q9GZV9 | 0.66 | 0.04 | 0.0000000 | blue | FGF-23 | NA | FGF-23 | 60 |
TNFSF14 | FALSE | 138_TNFSF14 | O43557 | 1.81 | 0.02 | 0.0000000 | blue | TNFSF14 | NA | TNFSF14 | 59 |
CCL11 | FALSE | 137_CCL11 | P51671 | 2.09 | 0.02 | 0.0000000 | blue | CCL11 | CCL11 | CCL11 | 58 |
MCP.4 | FALSE | 136_MCP-4 | Q99616 | 0.56 | 0.04 | 0.0000000 | blue | MCP-4 | CCL13 | CCL13 | 57 |
TGF.alpha | FALSE | 135_TGF-alpha | P01135 | 0.07 | 0.02 | 0.0000000 | blue | TGF-alpha | NA | TGF-alpha | 56 |
IL.18 | FALSE | 133_IL-18 | Q14116 | 2.79 | 0.02 | 0.0000000 | blue | IL-18 | NA | IL-18 | 55 |
SCF | FALSE | 132_SCF | P21583 | 2.30 | 0.02 | 0.0000000 | blue | SCF | NA | SCF | 54 |
CD6 | FALSE | 131_CD6 | Q8WWJ7 | 1.78 | 0.04 | 0.0000000 | blue | CD6 | NA | CD6 | 53 |
CCL4 | FALSE | 130_CCL4 | P13236 | 2.15 | 0.02 | 0.0000000 | blue | CCL4 | NA | CCL4 | 52 |
CXCL1 | FALSE | 128_CXCL1 | P09341 | 3.45 | 0.02 | 0.0000000 | blue | CXCL1 | NA | CXCL1 | 51 |
OSM | FALSE | 126_OSM | P13725 | 1.32 | 0.06 | 0.0000000 | blue | OSM | NA | OSM | 50 |
CST5 | FALSE | 123_CST5 | P28325 | 4.34 | 0.04 | 0.0000000 | blue | CST5 | NA | CST5 | 49 |
CXCL9 | FALSE | 122_CXCL9 | Q07325 | 1.04 | 0.02 | 0.0000000 | blue | CXCL9 | NA | CXCL9 | 48 |
TRAIL | FALSE | 120_TRAIL | P50591 | 1.22 | 0.02 | 0.0000000 | blue | TRAIL | NA | TRAIL | 47 |
CXCL11 | FALSE | 117_CXCL11 | O14625 | 1.40 | 0.02 | 0.0000000 | blue | CXCL11 | NA | CXCL11 | 46 |
MCP.1 | FALSE | 115_MCP-1 | P13500 | 1.90 | 0.02 | 0.0000000 | blue | MCP-1 | CCL2 | CCL2 | 45 |
uPA | FALSE | 112_uPA | P00749 | 1.75 | 0.02 | 0.0000000 | blue | uPA | NA | uPA | 44 |
LAP.TGF.beta.1 | FALSE | 111_LAP TGF-beta-1 | P01137 | 0.86 | 0.02 | 0.0000000 | blue | LAP TGF-beta-1 | NA | LAP TGF-beta-1 | 43 |
OPG | FALSE | 110_OPG | O00300 | 1.81 | 0.02 | 0.0000000 | blue | OPG | NA | OPG | 42 |
CD244 | FALSE | 108_CD244 | Q9BZW8 | 1.09 | 0.02 | 0.0000000 | blue | CD244 | NA | CD244 | 41 |
CDCP1 | FALSE | 107_CDCP1 | Q9H5V8 | 0.21 | 0.04 | 0.0000000 | blue | CDCP1 | NA | CDCP1 | 40 |
VEGF.A | FALSE | 102_VEGF-A | P15692 | 3.15 | 0.02 | 0.0000000 | blue | VEGF_A | NA | VEGF_A | 39 |
MIP.1.alpha | FALSE | 166_MIP-1 alpha | P10147 | 1.92 | 0.06 | 0.0203998 | blue | MIP-1 alpha | NA | MIP-1 alpha | 38 |
MMP.1 | FALSE | 142_MMP-1 | P03956 | 1.89 | 0.06 | 0.0407997 | blue | MMP-1 | NA | MMP-1 | 37 |
SIRT2 | FALSE | 172_SIRT2 | Q8IXJ6 | 1.52 | 1.00 | 0.0815993 | blue | SIRT2 | NA | SIRT2 | 36 |
IL.8 | FALSE | 101_IL-8 | P10145 | 3.17 | 0.25 | 0.2447980 | blue | IL-8 | NA | IL-8 | 35 |
IL.7 | FALSE | 109_IL-7 | P13232 | 1.39 | 1.43 | 0.7547940 | blue | IL-7 | NA | IL-7 | 34 |
SLAMF1 | FALSE | 134_SLAMF1 | Q13291 | 1.55 | 2.13 | 1.5299878 | blue | SLAMF1 | NA | SLAMF1 | 33 |
EN.RAGE | FALSE | 175_EN-RAGE | P80511 | 1.57 | 3.28 | 3.5291718 | blue | EN-RAGE | NA | EN-RAGE | 32 |
Beta.NGF | FALSE | 153_Beta-NGF | P01138 | 1.23 | 3.96 | 4.2227662 | blue | Beta-NGF | NA | Beta-NGF | 31 |
CCL28 | FALSE | 173_CCL28 | Q9NRJ3 | 0.92 | 5.49 | 5.2835577 | red | CCL28 | NA | CCL28 | 30 |
IL.10 | FALSE | 162_IL-10 | P22301 | 1.50 | 7.71 | 7.0583435 | blue | IL-10 | NA | IL-10 | 29 |
AXIN1 | FALSE | 118_AXIN1 | O15169 | 1.30 | 13.04 | 10.8731130 | red | AXIN1 | NA | AXIN1 | 28 |
NT.3 | FALSE | 188_NT-3 | P20783 | 0.85 | 12.77 | 13.2394941 | blue | NT-3 | NA | NT-3 | 27 |
FGF.5 | FALSE | 141_FGF-5 | Q8NF90 | 1.00 | 31.71 | 30.3957568 | blue | FGF-5 | NA | FGF-5 | 26 |
ST1A1 | FALSE | 191_ST1A1 | P50225 | 1.34 | 32.68 | 31.2525500 | blue | ST1A1 | NA | ST1A1 | 25 |
GDNF | FALSE | 106_GDNF | P39905 | 1.13 | 43.26 | 42.4520604 | blue | hGDNF | NA | hGDNF | 24 |
IL.10RA | FALSE | 140_IL-10RA | Q13651 | 1.14 | 53.30 | 53.9779682 | red | IL-10RA | NA | IL-10RA | 23 |
IL.6 | FALSE | 113_IL-6 | P05231 | 1.97 | 69.06 | 68.6250510 | blue | IL-6 | NA | IL-6 | 22 |
IL.5 | FALSE | 193_IL-5 | P05113 | 1.55 | 78.21 | 77.8457772 | red | IL-5 | NA | IL-5 | 21 |
MCP.3 | FALSE | 105_MCP-3 | P80098 | 1.31 | 79.30 | 79.0697674 | blue | MCP-3 | CCL7 | CCL7 | 20 |
IL.17C | FALSE | 114_IL-17C | Q9P0M4 | 1.28 | 83.07 | 83.3741330 | blue | IL-17C | NA | IL-17C | 19 |
IL.17A | FALSE | 116_IL-17A | Q16552 | 0.97 | 84.73 | 84.7001224 | red | IL-17A | NA | IL-17A | 18 |
IL.4 | FALSE | 180_IL-4 | P05112 | 1.11 | 93.91 | 94.4104447 | red | IL-4 | NA | IL-4 | 17 |
LIF | FALSE | 181_LIF | P15018 | 1.45 | 94.90 | 94.8184415 | red | LIF | NA | LIF | 16 |
TNF | FALSE | 163_TNF | P01375 | 1.19 | 95.22 | 95.1244390 | red | TNF | NA | TNF | 15 |
IL.20RA | FALSE | 121_IL-20RA | Q9UHF4 | 0.79 | 95.16 | 95.7772338 | red | IL-20RA | NA | IL-20RA | 14 |
ARTN | FALSE | 160_ARTN | Q5T4W7 | 0.73 | 95.84 | 95.9404325 | red | ARTN | NA | ARTN | 13 |
IL.15RA | FALSE | 148_IL-15RA | Q13261 | 1.18 | 96.10 | 96.1648307 | blue | IL-15RA | NA | IL-15RA | 12 |
IL.13 | FALSE | 159_IL-13 | P35225 | 1.71 | 96.51 | 96.5524276 | red | IL-13 | NA | IL-13 | 11 |
IL.20 | FALSE | 171_IL-20 | Q9NYY1 | 1.30 | 96.72 | 96.7156263 | red | IL-20 | NA | IL-20 | 10 |
IL.24 | FALSE | 158_IL-24 | Q13007 | 1.95 | 97.13 | 97.0828233 | red | IL-24 | NA | IL-24 | 9 |
IL.2RB | FALSE | 124_IL-2RB | P14784 | 1.47 | 97.11 | 97.1032232 | red | IL-2RB | NA | IL-2RB | 8 |
NRTN | FALSE | 182_NRTN | Q99748 | 1.39 | 97.11 | 97.1236230 | red | NRTN | NA | NRTN | 7 |
IL.33 | FALSE | 177_IL-33 | O95760 | 1.17 | 98.54 | 98.5516116 | red | IL-33 | NA | IL-33 | 6 |
TSLP | FALSE | 129_TSLP | Q969D9 | 1.80 | 99.49 | 99.4900041 | red | TSLP | NA | TSLP | 5 |
IFN.gamma | FALSE | 178_IFN-gamma | P01579 | 1.40 | 99.53 | 99.5308038 | red | IFN-gamma | NA | IFN-gamma | 4 |
IL.22.RA1 | FALSE | 150_IL-22 RA1 | Q8N6P7 | 1.79 | 99.61 | 99.6124031 | red | IL-22RA1 | NA | IL-22RA1 | 3 |
IL.2 | FALSE | 127_IL-2 | P60568 | 0.93 | 99.86 | 99.8776010 | red | IL-2 | NA | IL-2 | 2 |
IL.1.alpha | FALSE | 125_IL-1 alpha | P01583 | 4.76 | 99.88 | 99.8776010 | blue | IL-1 alpha | NA | IL-1 alpha | 1 |
8 maEndtoEnd
Web: https://bioconductor.org/packages/release/workflows/html/maEndToEnd.html.
Examples can be found on PCA, heatmap, normalisation, linear models, and enrichment analysis from this Bioconductor package.