es_pkgs <- c("Biobase", "arrayQualityMetrics", "dplyr", "knitr", "mclust", "pQTLtools", "rgl", "scatterplot3d")
se_pkgs <- c("BiocGenerics","GenomicRanges","IRanges","MsCoreUtils","S4Vectors","SummarizedExperiment","impute")
pkgs <- c(es_pkgs,se_pkgs)
for (p in pkgs) if (length(grep(paste("^package:", p, "$", sep=""), search())) == 0) {
if (!requireNamespace(p)) warning(paste0("This vignette needs package `", p, "'; please install"))
}
invisible(suppressMessages(lapply(pkgs, require, character.only = TRUE)))
1 ExpressionSet
We start with Bioconductor/Biobase’s ExpressionSet example and finish with a real application.
dataDirectory <- system.file("extdata", package="Biobase")
exprsFile <- file.path(dataDirectory, "exprsData.txt")
exprs <- as.matrix(read.table(exprsFile, header=TRUE, sep="\t", row.names=1, as.is=TRUE))
pDataFile <- file.path(dataDirectory, "pData.txt")
pData <- read.table(pDataFile, row.names=1, header=TRUE, sep="\t")
all(rownames(pData)==colnames(exprs))
metadata <- data.frame(labelDescription=c("Patient gender",
"Case/control status",
"Tumor progress on XYZ scale"),
row.names=c("gender", "type", "score"))
phenoData <- Biobase::AnnotatedDataFrame(data=pData, varMetadata=metadata)
experimentData <- Biobase::MIAME(name="Pierre Fermat",
lab="Francis Galton Lab",
contact="pfermat@lab.not.exist",
title="Smoking-Cancer Experiment",
abstract="An example ExpressionSet",
url="www.lab.not.exist",
other=list(notes="Created from text files"))
exampleSet <- pQTLtools::make_ExpressionSet(exprs,phenoData,experimentData=experimentData,
annotation="hgu95av2")
data(sample.ExpressionSet, package="Biobase")
identical(exampleSet,sample.ExpressionSet)
1.1 data.frame
The great benefit is to use the object directly as a data.frame.
lm.result <- Biobase::esApply(exampleSet,1,function(x) lm(score~gender+x))
beta.x <- unlist(lapply(lapply(lm.result,coef),"[",3))
beta.x[1]
#> AFFX-MurIL2_at.x
#> -0.0001907472
lm(score~gender+AFFX.MurIL2_at,data=exampleSet)
#>
#> Call:
#> lm(formula = score ~ gender + AFFX.MurIL2_at, data = exampleSet)
#>
#> Coefficients:
#> (Intercept) genderMale AFFX.MurIL2_at
#> 0.5531725 0.0098932 -0.0001907
1.2 Composite plots
We wish to examine the distribution of each feature via histogram, scatter and boxplot.
One could resort to esApply()
for its simplicity as before
invisible(Biobase::esApply(exampleSet[1:2],1,function(x)
{par(mfrow=c(3,1));boxplot(x);hist(x);plot(x)}
))
but it is nicer to add feature name in the title.
par(mfrow=c(1,3))
f <- Biobase::featureNames(exampleSet[1:2])
invisible(sapply(f,function(x) {
d <- t(Biobase::exprs(exampleSet[x]))
fn <- Biobase::featureNames(exampleSet[x])
hist(d,main="",xlab=fn); plot(d, ylab=fn); boxplot(d,ylab=fn)
}
)
)
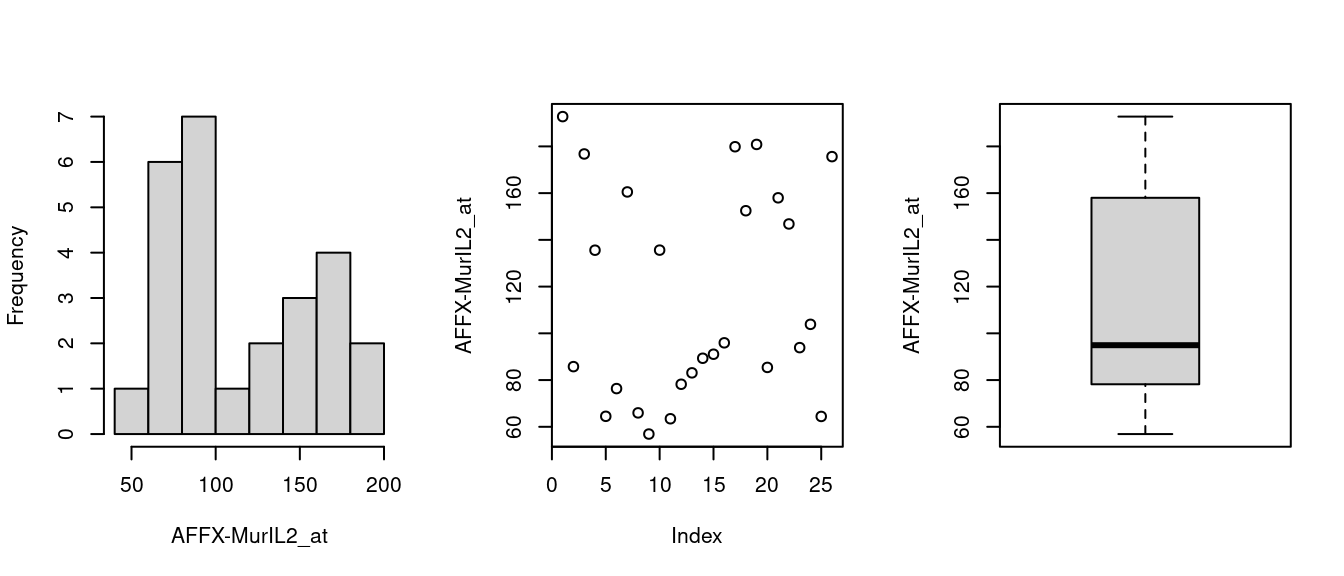
Figure 1.1: Histogram, scatter & boxplots
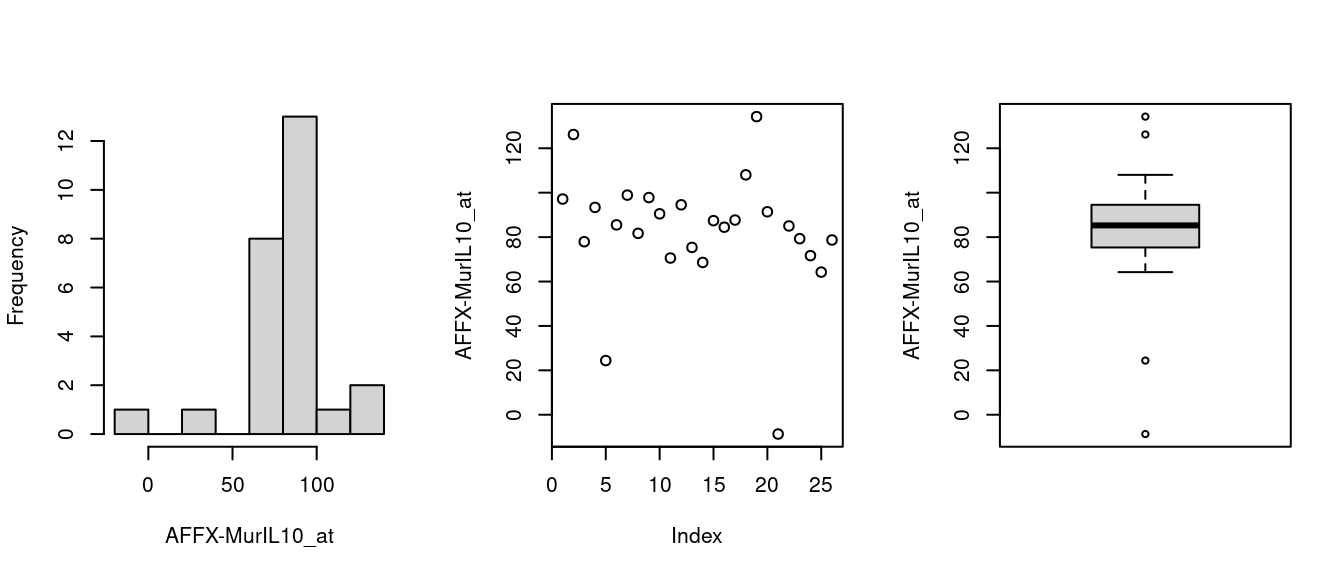
Figure 1.2: Histogram, scatter & boxplots
where the expression set is indexed using feature name(s).
1.4 Clustering
We employ model-based clustering absed on principal compoents to see potential groupings in the data,
pca <- prcomp(na.omit(t(Biobase::exprs(exampleSet))), rank=10, scale=TRUE)
pc1pc2pc3 <- with(pca,x)[,1:3]
mc <- mclust::Mclust(pc1pc2pc3,G=3)
with(mc, {
cols <- c("blue","red", "purple")
s3d <- scatterplot3d::scatterplot3d(with(pca,x[,c(2,1,3)]),
color=cols[classification],
pch=16,
type="h",
main="Plot of the PC1, PC2 and PC3")
s3d.coords <- s3d$xyz.convert(with(pca,x[,c(2,1,3)]))
text(s3d.coords$x,
s3d.coords$y,
cex = 1.2,
col = cols[classification],
labels = row.names(pc1pc2pc3),
pos = 4)
legend("right", legend=levels(as.factor(classification)), col=cols[classification], pch=16)
rgl::open3d(width = 500, height = 500)
rgl::plot3d(with(pca,x[,c(2,1,3)]),cex=1.2,col=cols[classification],size=5)
rgl::text3d(with(pca,x[,c(2,1,3)]),cex=1.2,col=cols[classification],texts=row.names(pc1pc2pc3))
htmlwidgets::saveWidget(rgl::rglwidget(), file = "mcpca3d.html")
})
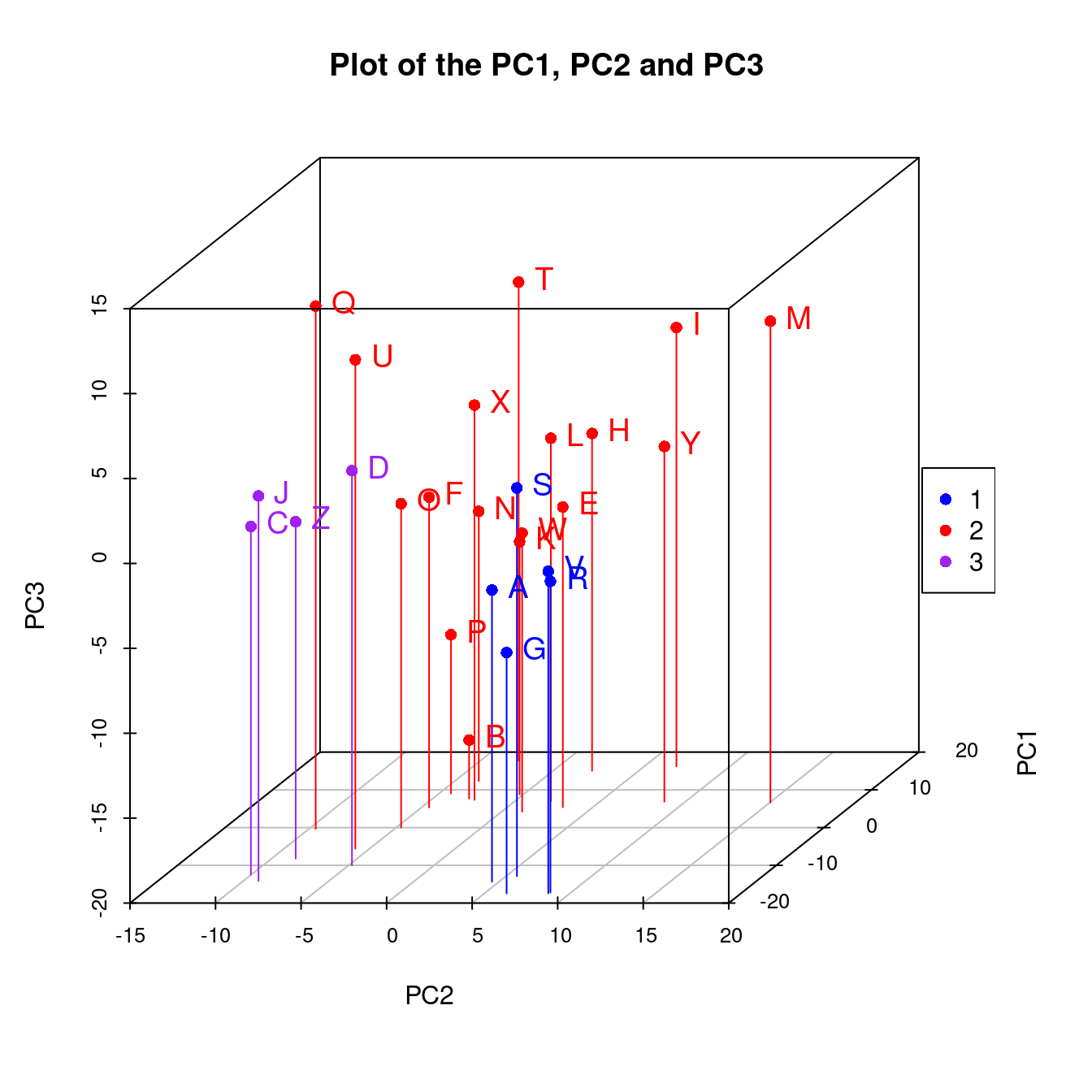
Figure 1.3: Three-group Clustering
#> Warning in par3d(userMatrix = structure(c(1, 0, 0, 0, 0, 0.342020143325668, :
#> parameter "width" cannot be set
#> Warning in par3d(userMatrix = structure(c(1, 0, 0, 0, 0, 0.342020143325668, :
#> parameter "height" cannot be set
An interactive version is also available,
1.5 Data transformation
Suppose we wish use log2 for those greater than zero but set those negative values to be missing.
1.6 Limit of detection (LOD)
We generate a lod.max
~ U[0,1] variable to experiment
Biobase::fData(exampleSet)
#> data frame with 0 columns and 500 rows
Biobase::fData(exampleSet)$lod.max <- apply(Biobase::exprs(exampleSet),1,quantile,runif(nrow(exampleSet)))
lod <- pQTLtools::get.prop.below.LLOD(exampleSet)
x <- dplyr::arrange(Biobase::fData(lod),desc(pc.belowLOD.new))
knitr::kable(head(lod))
AFFX.MurIL2_at | AFFX.MurIL10_at | AFFX.MurIL4_at | AFFX.MurFAS_at | AFFX.BioB.5_at | AFFX.BioB.M_at | gender | type | score | |
---|---|---|---|---|---|---|---|---|---|
A | 192.7420 | 97.13700 | 45.81920 | 22.54450 | 96.7875 | 89.0730 | Female | Control | 0.75 |
B | 85.7533 | 126.19600 | 8.83135 | 3.60093 | 30.4380 | 25.8461 | Male | Case | 0.40 |
C | 176.7570 | 77.92160 | 33.06320 | 14.68830 | 46.1271 | 57.2033 | Male | Control | 0.73 |
D | 135.5750 | 93.37130 | 28.70720 | 12.33970 | 70.9319 | 69.9766 | Male | Case | 0.42 |
E | 64.4939 | 24.39860 | 5.94492 | 36.86630 | 56.1744 | 49.5822 | Female | Case | 0.93 |
F | 76.3569 | 85.50880 | 28.29250 | 11.25680 | 42.6756 | 26.1262 | Male | Control | 0.22 |
G | 160.5050 | 98.90860 | 30.96940 | 23.00340 | 86.5156 | 75.0083 | Male | Case | 0.96 |
H | 65.9631 | 81.69320 | 14.79230 | 16.21340 | 30.7927 | 42.3352 | Male | Case | 0.79 |
I | 56.9039 | 97.80150 | 14.23990 | 12.03750 | 19.7183 | 41.1207 | Female | Case | 0.37 |
J | 135.6080 | 90.48380 | 34.48740 | 4.54978 | 46.3520 | 91.5307 | Male | Control | 0.63 |
K | 63.4432 | 70.57330 | 20.35210 | 8.51782 | 39.1326 | 39.9136 | Male | Case | 0.26 |
L | 78.2126 | 94.54180 | 14.15540 | 27.28520 | 41.7698 | 49.8397 | Female | Control | 0.36 |
M | 83.0943 | 75.34550 | 20.62510 | 10.16160 | 80.2197 | 63.4794 | Male | Case | 0.41 |
N | 89.3372 | 68.58270 | 15.92310 | 20.24880 | 36.4903 | 24.7007 | Male | Case | 0.80 |
O | 91.0615 | 87.40500 | 20.15790 | 15.78490 | 36.4021 | 47.4641 | Female | Case | 0.10 |
P | 95.9377 | 84.45810 | 27.81390 | 14.32760 | 35.3054 | 47.3578 | Female | Control | 0.41 |
Q | 179.8450 | 87.68060 | 32.79110 | 15.94880 | 58.6239 | 58.1331 | Female | Case | 0.16 |
R | 152.4670 | 108.03200 | 33.52920 | 14.67530 | 114.0620 | 104.1220 | Male | Control | 0.72 |
S | 180.8340 | 134.26300 | 19.81720 | -7.91911 | 93.4402 | 115.8310 | Male | Case | 0.17 |
T | 85.4146 | 91.40310 | 20.41900 | 12.88750 | 22.5168 | 58.1224 | Female | Case | 0.74 |
U | 157.9890 | -8.68811 | 26.87200 | 11.91860 | 48.6462 | 73.4221 | Male | Control | 0.35 |
V | 146.8000 | 85.02120 | 31.14880 | 12.83240 | 90.2215 | 64.6066 | Female | Control | 0.77 |
W | 93.8829 | 79.29980 | 22.34200 | 11.13900 | 42.0053 | 40.3068 | Male | Control | 0.27 |
X | 103.8550 | 71.65520 | 19.01350 | 7.55564 | 57.5738 | 41.8209 | Male | Control | 0.98 |
Y | 64.4340 | 64.23690 | 12.16860 | 19.98490 | 44.8216 | 46.1087 | Female | Case | 0.94 |
Z | 175.6150 | 78.70680 | 17.37800 | 8.96849 | 61.7044 | 49.4122 | Female | Case | 0.32 |
plot(x[,2], main="Random quantile cutoff", ylab="<lod%")
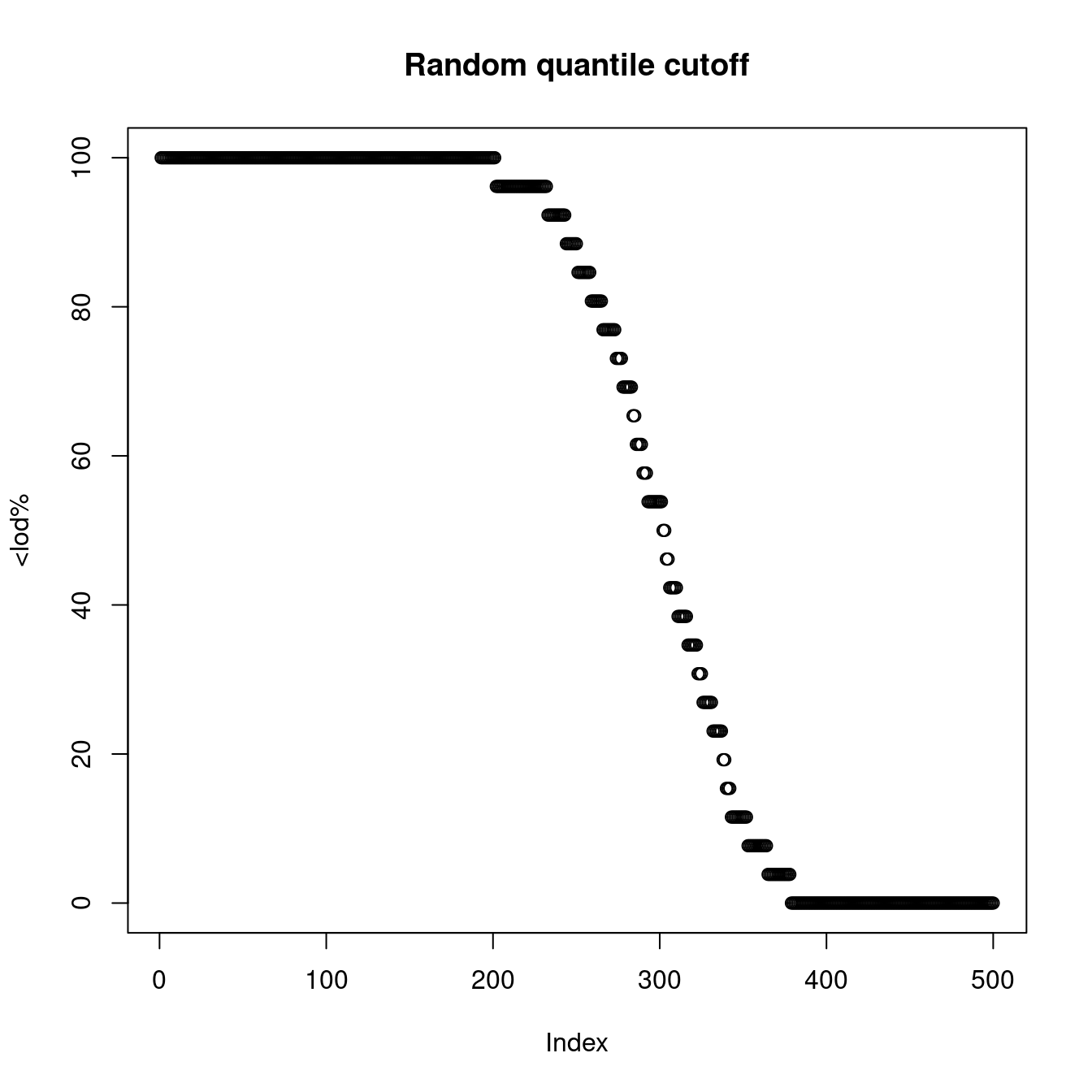
Figure 1.4: LOD based on a random cutoff
The quantity has been shown to have a big impact on protein abundance and therefore pQTL detection as is shown with a real example.
rm(list=ls())
dir <- "~/rds/post_qc_data/interval/phenotype/olink_proteomics/post-qc/"
eset <- readRDS(paste0(dir,"eset.inf1.flag.out.outlier.in.rds"))
x <- pQTLtools::get.prop.below.LLOD(eset)
annot <- Biobase::fData(x)
annot$MissDataProp <- as.numeric(gsub("\\%$", "", annot$MissDataProp))
plot(annot$MissDataProp, annot$pc.belowLOD.new, xlab="% <LLOD in Original",
ylab="% <LLOD in post QC dataset", pch=19)
INF <- Sys.getenv("INF")
np <- read.table(paste(INF, "work", "INF1.merge.nosig", sep="/"), header=FALSE,
col.names = c("prot", "uniprot"))
kable(np, caption="Proteins with no pQTL")
annot$pQTL <- rep(NA, nrow(annot))
no.pQTL.ind <- which(annot$uniprot.id %in% np$uniprot)
annot$pQTL[no.pQTL.ind] <- "red"
annot$pQTL[-no.pQTL.ind] <- "blue"
annot <- annot[order(annot$pc.belowLOD.new, decreasing = TRUE),]
annot <- annot[-grep("^BDNF$", annot$ID),]
saveRDS(annot,file=file.path("~","pQTLtools","tests","annot.RDS"))
annot <- readRDS(file.path(find.package("pQTLtools"),"tests","annot.RDS")) %>%
dplyr::left_join(pQTLdata::inf1[c("prot","target.short","alt_name")],by=c("ID"="prot")) %>%
dplyr::mutate(prot=if_else(is.na(alt_name),target.short,alt_name),order=1:n()) %>%
dplyr::arrange(desc(order))
xtick <- seq(1, nrow(annot))
attach(annot)
par(mar=c(10,5,1,1))
plot(100-pc.belowLOD.new,cex=2,pch=19,col=pQTL,xaxt="n",xlab="",ylab="",cex.axis=0.8)
text(66,16,"IL-17C",offset=0,pos=2,cex=1.5,font=2,srt=0)
arrows(67,16,71,16,lwd=2)
axis(1, at=xtick, labels=prot, lwd.tick=0.5, lwd=0, las=2, hadj=1, cex.axis=0.8)
mtext("% samples above LLOD",side=2,line=2.5,cex=1.2)
mtext("Ordered protein",side=1,line=6.5,cex=1.2,font=1)
legend(x=1,y=25,c("without pQTL","with pQTL"),box.lwd=0,cex=2,col=c("red","blue"),pch=19)
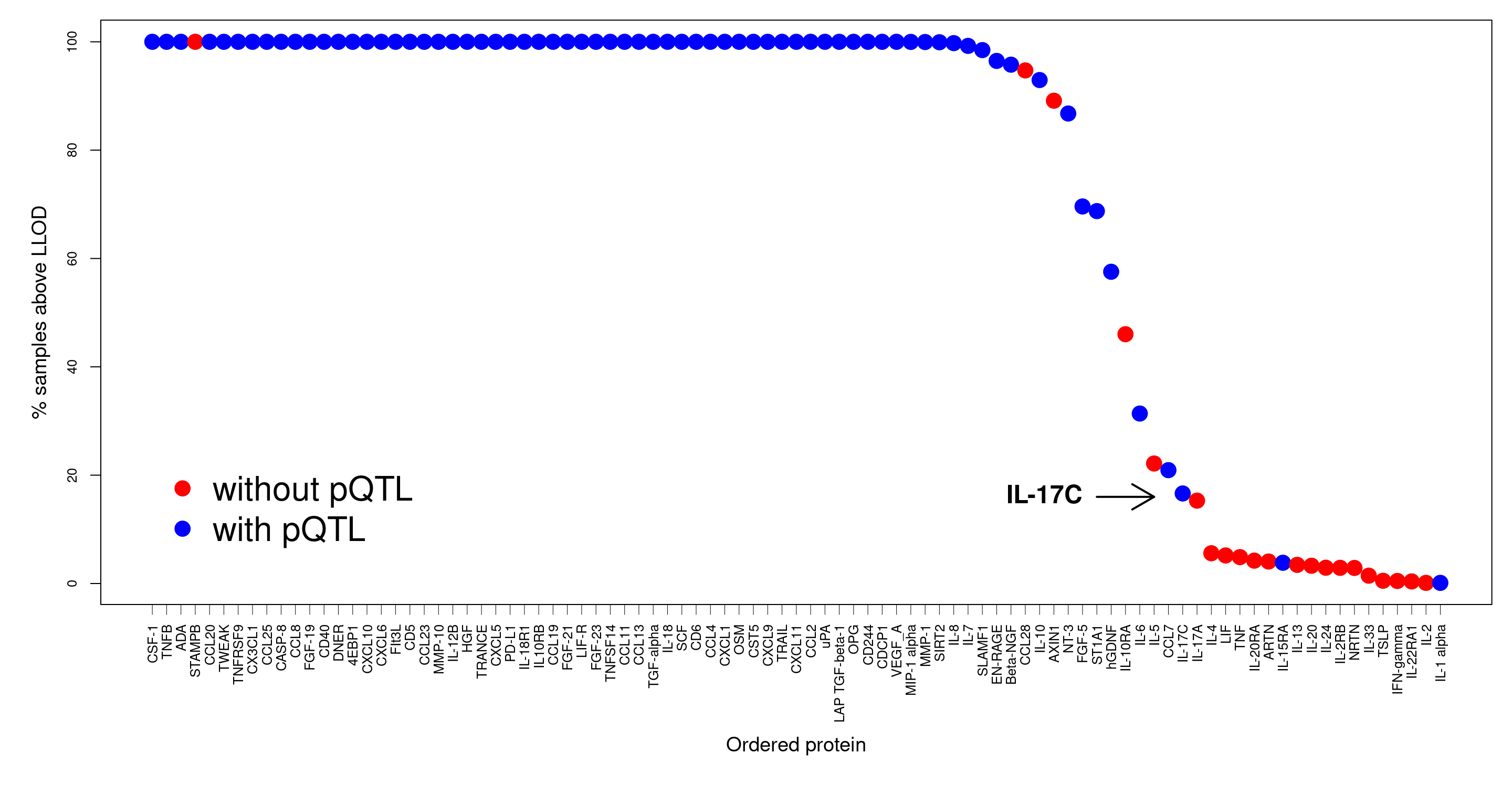
Figure 1.5: LOD in SCALLOP-INF/INTERVAL
ID | dichot | olink.id | uniprot.id | lod.max | MissDataProp | pc.belowLOD.new | pQTL | target.short | alt_name | prot | order |
---|---|---|---|---|---|---|---|---|---|---|---|
CSF.1 | FALSE | 196_CSF-1 | P09603 | 1.02 | 0.02 | 0.0000000 | blue | CSF-1 | NA | CSF-1 | 91 |
TNFB | FALSE | 195_TNFB | P01374 | 1.10 | 0.04 | 0.0000000 | blue | TNFB | NA | TNFB | 90 |
ADA | FALSE | 194_ADA | P00813 | 1.80 | 0.04 | 0.0000000 | blue | ADA | NA | ADA | 89 |
STAMPB | FALSE | 192_STAMPB | O95630 | 1.40 | 0.06 | 0.0000000 | red | STAMPB | NA | STAMPB | 88 |
CCL20 | FALSE | 190_CCL20 | P78556 | 1.42 | 0.02 | 0.0000000 | blue | CCL20 | NA | CCL20 | 87 |
TWEAK | FALSE | 189_TWEAK | O43508 | 1.78 | 0.02 | 0.0000000 | blue | TWEAK | NA | TWEAK | 86 |
TNFRSF9 | FALSE | 187_TNFRSF9 | Q07011 | 1.86 | 0.02 | 0.0000000 | blue | TNFRSF9 | NA | TNFRSF9 | 85 |
CX3CL1 | FALSE | 186_CX3CL1 | P78423 | 1.76 | 0.02 | 0.0000000 | blue | CX3CL1 | NA | CX3CL1 | 84 |
CCL25 | FALSE | 185_CCL25 | O15444 | 1.32 | 0.02 | 0.0000000 | blue | CCL25 | NA | CCL25 | 83 |
CASP.8 | FALSE | 184_CASP-8 | Q14790 | 1.38 | 0.04 | 0.0000000 | blue | CASP-8 | NA | CASP-8 | 82 |
MCP.2 | FALSE | 183_MCP-2 | P80075 | 2.10 | 0.02 | 0.0000000 | blue | MCP-2 | CCL8 | CCL8 | 81 |
FGF.19 | FALSE | 179_FGF-19 | O95750 | 1.25 | 0.02 | 0.0000000 | blue | FGF-19 | NA | FGF-19 | 80 |
CD40 | FALSE | 176_CD40 | P25942 | 1.91 | 0.04 | 0.0000000 | blue | CD40 | NA | CD40 | 79 |
DNER | FALSE | 174_DNER | Q8NFT8 | 1.63 | 0.02 | 0.0000000 | blue | DNER | NA | DNER | 78 |
4E.BP1 | FALSE | 170_4E-BP1 | Q13541 | 1.04 | 0.10 | 0.0000000 | blue | 4EBP1 | NA | 4EBP1 | 77 |
CXCL10 | FALSE | 169_CXCL10 | P02778 | 2.03 | 0.02 | 0.0000000 | blue | CXCL10 | NA | CXCL10 | 76 |
CXCL6 | FALSE | 168_CXCL6 | P80162 | 1.80 | 0.02 | 0.0000000 | blue | CXCL6 | CXCL6 | CXCL6 | 75 |
Flt3L | FALSE | 167_Flt3L | P49771 | 2.12 | 0.02 | 0.0000000 | blue | FIt3L | NA | FIt3L | 74 |
CD5 | FALSE | 165_CD5 | P06127 | 1.42 | 0.02 | 0.0000000 | blue | CD5 | NA | CD5 | 73 |
CCL23 | FALSE | 164_CCL23 | P55773 | 2.10 | 0.02 | 0.0000000 | blue | CCL23 | NA | CCL23 | 72 |
MMP.10 | FALSE | 161_MMP-10 | P09238 | 1.56 | 0.02 | 0.0000000 | blue | MMP-10 | NA | MMP-10 | 71 |
IL.12B | FALSE | 157_IL-12B | P29460 | 0.85 | 0.02 | 0.0000000 | blue | IL-12B | NA | IL-12B | 70 |
HGF | FALSE | 156_HGF | P14210 | 1.77 | 0.02 | 0.0000000 | blue | HGF | NA | HGF | 69 |
TRANCE | FALSE | 155_TRANCE | O14788 | 1.51 | 0.04 | 0.0000000 | blue | TRANCE | NA | TRANCE | 68 |
CXCL5 | FALSE | 154_CXCL5 | P42830 | 1.92 | 0.02 | 0.0000000 | blue | CXCL5 | NA | CXCL5 | 67 |
PD.L1 | FALSE | 152_PD-L1 | Q9NZQ7 | 2.14 | 0.04 | 0.0000000 | blue | PD-L1 | NA | PD-L1 | 66 |
IL.18R1 | FALSE | 151_IL-18R1 | Q13478 | 1.21 | 0.02 | 0.0000000 | blue | IL-18R1 | NA | IL-18R1 | 65 |
IL.10RB | FALSE | 149_IL-10RB | Q08334 | 1.72 | 0.02 | 0.0000000 | blue | IL10RB | NA | IL10RB | 64 |
CCL19 | FALSE | 145_CCL19 | Q99731 | 2.13 | 0.02 | 0.0000000 | blue | CCL19 | NA | CCL19 | 63 |
FGF.21 | FALSE | 144_FGF-21 | Q9NSA1 | 1.14 | 0.02 | 0.0000000 | blue | FGF-21 | NA | FGF-21 | 62 |
LIF.R | FALSE | 143_LIF-R | P42702 | 2.08 | 0.04 | 0.0000000 | blue | LIF-R | NA | LIF-R | 61 |
FGF.23 | FALSE | 139_FGF-23 | Q9GZV9 | 0.66 | 0.04 | 0.0000000 | blue | FGF-23 | NA | FGF-23 | 60 |
TNFSF14 | FALSE | 138_TNFSF14 | O43557 | 1.81 | 0.02 | 0.0000000 | blue | TNFSF14 | NA | TNFSF14 | 59 |
CCL11 | FALSE | 137_CCL11 | P51671 | 2.09 | 0.02 | 0.0000000 | blue | CCL11 | CCL11 | CCL11 | 58 |
MCP.4 | FALSE | 136_MCP-4 | Q99616 | 0.56 | 0.04 | 0.0000000 | blue | MCP-4 | CCL13 | CCL13 | 57 |
TGF.alpha | FALSE | 135_TGF-alpha | P01135 | 0.07 | 0.02 | 0.0000000 | blue | TGF-alpha | NA | TGF-alpha | 56 |
IL.18 | FALSE | 133_IL-18 | Q14116 | 2.79 | 0.02 | 0.0000000 | blue | IL-18 | NA | IL-18 | 55 |
SCF | FALSE | 132_SCF | P21583 | 2.30 | 0.02 | 0.0000000 | blue | SCF | NA | SCF | 54 |
CD6 | FALSE | 131_CD6 | Q8WWJ7 | 1.78 | 0.04 | 0.0000000 | blue | CD6 | NA | CD6 | 53 |
CCL4 | FALSE | 130_CCL4 | P13236 | 2.15 | 0.02 | 0.0000000 | blue | CCL4 | NA | CCL4 | 52 |
CXCL1 | FALSE | 128_CXCL1 | P09341 | 3.45 | 0.02 | 0.0000000 | blue | CXCL1 | NA | CXCL1 | 51 |
OSM | FALSE | 126_OSM | P13725 | 1.32 | 0.06 | 0.0000000 | blue | OSM | NA | OSM | 50 |
CST5 | FALSE | 123_CST5 | P28325 | 4.34 | 0.04 | 0.0000000 | blue | CST5 | NA | CST5 | 49 |
CXCL9 | FALSE | 122_CXCL9 | Q07325 | 1.04 | 0.02 | 0.0000000 | blue | CXCL9 | NA | CXCL9 | 48 |
TRAIL | FALSE | 120_TRAIL | P50591 | 1.22 | 0.02 | 0.0000000 | blue | TRAIL | NA | TRAIL | 47 |
CXCL11 | FALSE | 117_CXCL11 | O14625 | 1.40 | 0.02 | 0.0000000 | blue | CXCL11 | NA | CXCL11 | 46 |
MCP.1 | FALSE | 115_MCP-1 | P13500 | 1.90 | 0.02 | 0.0000000 | blue | MCP-1 | CCL2 | CCL2 | 45 |
uPA | FALSE | 112_uPA | P00749 | 1.75 | 0.02 | 0.0000000 | blue | uPA | NA | uPA | 44 |
LAP.TGF.beta.1 | FALSE | 111_LAP TGF-beta-1 | P01137 | 0.86 | 0.02 | 0.0000000 | blue | LAP TGF-beta-1 | NA | LAP TGF-beta-1 | 43 |
OPG | FALSE | 110_OPG | O00300 | 1.81 | 0.02 | 0.0000000 | blue | OPG | NA | OPG | 42 |
CD244 | FALSE | 108_CD244 | Q9BZW8 | 1.09 | 0.02 | 0.0000000 | blue | CD244 | NA | CD244 | 41 |
CDCP1 | FALSE | 107_CDCP1 | Q9H5V8 | 0.21 | 0.04 | 0.0000000 | blue | CDCP1 | NA | CDCP1 | 40 |
VEGF.A | FALSE | 102_VEGF-A | P15692 | 3.15 | 0.02 | 0.0000000 | blue | VEGF_A | NA | VEGF_A | 39 |
MIP.1.alpha | FALSE | 166_MIP-1 alpha | P10147 | 1.92 | 0.06 | 0.0203998 | blue | MIP-1 alpha | NA | MIP-1 alpha | 38 |
MMP.1 | FALSE | 142_MMP-1 | P03956 | 1.89 | 0.06 | 0.0407997 | blue | MMP-1 | NA | MMP-1 | 37 |
SIRT2 | FALSE | 172_SIRT2 | Q8IXJ6 | 1.52 | 1.00 | 0.0815993 | blue | SIRT2 | NA | SIRT2 | 36 |
IL.8 | FALSE | 101_IL-8 | P10145 | 3.17 | 0.25 | 0.2447980 | blue | IL-8 | NA | IL-8 | 35 |
IL.7 | FALSE | 109_IL-7 | P13232 | 1.39 | 1.43 | 0.7547940 | blue | IL-7 | NA | IL-7 | 34 |
SLAMF1 | FALSE | 134_SLAMF1 | Q13291 | 1.55 | 2.13 | 1.5299878 | blue | SLAMF1 | NA | SLAMF1 | 33 |
EN.RAGE | FALSE | 175_EN-RAGE | P80511 | 1.57 | 3.28 | 3.5291718 | blue | EN-RAGE | NA | EN-RAGE | 32 |
Beta.NGF | FALSE | 153_Beta-NGF | P01138 | 1.23 | 3.96 | 4.2227662 | blue | Beta-NGF | NA | Beta-NGF | 31 |
CCL28 | FALSE | 173_CCL28 | Q9NRJ3 | 0.92 | 5.49 | 5.2835577 | red | CCL28 | NA | CCL28 | 30 |
IL.10 | FALSE | 162_IL-10 | P22301 | 1.50 | 7.71 | 7.0583435 | blue | IL-10 | NA | IL-10 | 29 |
AXIN1 | FALSE | 118_AXIN1 | O15169 | 1.30 | 13.04 | 10.8731130 | red | AXIN1 | NA | AXIN1 | 28 |
NT.3 | FALSE | 188_NT-3 | P20783 | 0.85 | 12.77 | 13.2394941 | blue | NT-3 | NA | NT-3 | 27 |
FGF.5 | FALSE | 141_FGF-5 | Q8NF90 | 1.00 | 31.71 | 30.3957568 | blue | FGF-5 | NA | FGF-5 | 26 |
ST1A1 | FALSE | 191_ST1A1 | P50225 | 1.34 | 32.68 | 31.2525500 | blue | ST1A1 | NA | ST1A1 | 25 |
GDNF | FALSE | 106_GDNF | P39905 | 1.13 | 43.26 | 42.4520604 | blue | hGDNF | NA | hGDNF | 24 |
IL.10RA | FALSE | 140_IL-10RA | Q13651 | 1.14 | 53.30 | 53.9779682 | red | IL-10RA | NA | IL-10RA | 23 |
IL.6 | FALSE | 113_IL-6 | P05231 | 1.97 | 69.06 | 68.6250510 | blue | IL-6 | NA | IL-6 | 22 |
IL.5 | FALSE | 193_IL-5 | P05113 | 1.55 | 78.21 | 77.8457772 | red | IL-5 | NA | IL-5 | 21 |
MCP.3 | FALSE | 105_MCP-3 | P80098 | 1.31 | 79.30 | 79.0697674 | blue | MCP-3 | CCL7 | CCL7 | 20 |
IL.17C | FALSE | 114_IL-17C | Q9P0M4 | 1.28 | 83.07 | 83.3741330 | blue | IL-17C | NA | IL-17C | 19 |
IL.17A | FALSE | 116_IL-17A | Q16552 | 0.97 | 84.73 | 84.7001224 | red | IL-17A | NA | IL-17A | 18 |
IL.4 | FALSE | 180_IL-4 | P05112 | 1.11 | 93.91 | 94.4104447 | red | IL-4 | NA | IL-4 | 17 |
LIF | FALSE | 181_LIF | P15018 | 1.45 | 94.90 | 94.8184415 | red | LIF | NA | LIF | 16 |
TNF | FALSE | 163_TNF | P01375 | 1.19 | 95.22 | 95.1244390 | red | TNF | NA | TNF | 15 |
IL.20RA | FALSE | 121_IL-20RA | Q9UHF4 | 0.79 | 95.16 | 95.7772338 | red | IL-20RA | NA | IL-20RA | 14 |
ARTN | FALSE | 160_ARTN | Q5T4W7 | 0.73 | 95.84 | 95.9404325 | red | ARTN | NA | ARTN | 13 |
IL.15RA | FALSE | 148_IL-15RA | Q13261 | 1.18 | 96.10 | 96.1648307 | blue | IL-15RA | NA | IL-15RA | 12 |
IL.13 | FALSE | 159_IL-13 | P35225 | 1.71 | 96.51 | 96.5524276 | red | IL-13 | NA | IL-13 | 11 |
IL.20 | FALSE | 171_IL-20 | Q9NYY1 | 1.30 | 96.72 | 96.7156263 | red | IL-20 | NA | IL-20 | 10 |
IL.24 | FALSE | 158_IL-24 | Q13007 | 1.95 | 97.13 | 97.0828233 | red | IL-24 | NA | IL-24 | 9 |
IL.2RB | FALSE | 124_IL-2RB | P14784 | 1.47 | 97.11 | 97.1032232 | red | IL-2RB | NA | IL-2RB | 8 |
NRTN | FALSE | 182_NRTN | Q99748 | 1.39 | 97.11 | 97.1236230 | red | NRTN | NA | NRTN | 7 |
IL.33 | FALSE | 177_IL-33 | O95760 | 1.17 | 98.54 | 98.5516116 | red | IL-33 | NA | IL-33 | 6 |
TSLP | FALSE | 129_TSLP | Q969D9 | 1.80 | 99.49 | 99.4900041 | red | TSLP | NA | TSLP | 5 |
IFN.gamma | FALSE | 178_IFN-gamma | P01579 | 1.40 | 99.53 | 99.5308038 | red | IFN-gamma | NA | IFN-gamma | 4 |
IL.22.RA1 | FALSE | 150_IL-22 RA1 | Q8N6P7 | 1.79 | 99.61 | 99.6124031 | red | IL-22RA1 | NA | IL-22RA1 | 3 |
IL.2 | FALSE | 127_IL-2 | P60568 | 0.93 | 99.86 | 99.8776010 | red | IL-2 | NA | IL-2 | 2 |
IL.1.alpha | FALSE | 125_IL-1 alpha | P01583 | 4.76 | 99.88 | 99.8776010 | blue | IL-1 alpha | NA | IL-1 alpha | 1 |
1.7 maEndtoEnd
Web: https://bioconductor.org/packages/release/workflows/html/maEndToEnd.html.
Examples can be found on PCA, heatmap, normalisation, linear models, and enrichment analysis from this Bioconductor package.
2 SummarizedExperiment
This is a more modern construct. Based on the documentation example, ranged summarized experiment (rse) and imputation are shown below.
set.seed(123)
nrows <- 20
ncols <- 4
counts <- matrix(runif(nrows * ncols, 1, 1e4), nrows)
missing_indices <- sample(length(counts), size = 5, replace = FALSE)
counts[missing_indices] <- NA
rowRanges <- GenomicRanges::GRanges(rep(c("chr1", "chr2"), c(1, 3) * nrows / 4),
IRanges::IRanges(floor(runif(nrows, 1e5, 1e6)), width=ncols),
strand=sample(c("+", "-"), nrows, TRUE),
feature_id=sprintf("ID%03d", 1:nrows))
colData <- S4Vectors::DataFrame(Treatment=rep(c("ChIP", "Input"), ncols/2),
row.names=LETTERS[1:ncols])
rse <- SummarizedExperiment::SummarizedExperiment(assays=S4Vectors::SimpleList(counts=counts),
rowRanges=rowRanges, colData=colData)
SummarizedExperiment::assay(rse)
#> A B C D
#> [1,] 2876.4876 8895.5036 1428.8574 6651.486831
#> [2,] 7883.2630 6928.3413 4146.0488 949.311769
#> [3,] 4090.3602 6405.4276 4137.8295 3840.312408
#> [4,] 8830.2910 9942.7035 3689.0857 2744.562062
#> [5,] 9404.7324 6557.4023 1525.2950 8146.585749
#> [6,] NA 7085.5962 1388.9218 4485.714898
#> [7,] 5281.5268 5441.1162 2331.1080 8100.833466
#> [8,] 8924.2980 5941.8261 4660.1585 8124.082706
#> [9,] 5514.7987 2892.3082 2660.4604 7943.628869
#> [10,] 4566.6907 1471.9894 NA 4398.877044
#> [11,] 9568.3766 NA 459.2658 7544.997111
#> [12,] 4533.8882 9023.0882 4422.5585 NA
#> [13,] 6776.0288 6907.3621 7989.4495 7102.113831
#> [14,] 5726.7614 7954.8787 1219.8707 7.247108
#> [15,] 1030.1439 247.1122 5609.9189 4753.690424
#> [16,] 8998.3499 4778.4819 2066.1074 2201.968733
#> [17,] 2461.6313 7584.8369 1276.1890 3798.785561
#> [18,] 421.5533 2164.8630 7533.3253 6128.097262
#> [19,] 3279.8793 NA 8950.5585 3518.627295
#> [20,] 9545.0820 2317.0262 3745.2533 1112.243108
imputed <- MsCoreUtils::impute_knn(as.matrix(SummarizedExperiment::assay(rse)),2)
imputed_counts <- MsCoreUtils::impute_RF(as.matrix(SummarizedExperiment::assay(rse)),2)
imputed-imputed_counts
#> A B C D
#> [1,] 0.000 0.0000 0.000 0.000
#> [2,] 0.000 0.0000 0.000 0.000
#> [3,] 0.000 0.0000 0.000 0.000
#> [4,] 0.000 0.0000 0.000 0.000
#> [5,] 0.000 0.0000 0.000 0.000
#> [6,] 2559.339 0.0000 0.000 0.000
#> [7,] 0.000 0.0000 0.000 0.000
#> [8,] 0.000 0.0000 0.000 0.000
#> [9,] 0.000 0.0000 0.000 0.000
#> [10,] 0.000 0.0000 1791.909 0.000
#> [11,] 0.000 -709.2786 0.000 0.000
#> [12,] 0.000 0.0000 0.000 2467.614
#> [13,] 0.000 0.0000 0.000 0.000
#> [14,] 0.000 0.0000 0.000 0.000
#> [15,] 0.000 0.0000 0.000 0.000
#> [16,] 0.000 0.0000 0.000 0.000
#> [17,] 0.000 0.0000 0.000 0.000
#> [18,] 0.000 0.0000 0.000 0.000
#> [19,] 0.000 1298.3456 0.000 0.000
#> [20,] 0.000 0.0000 0.000 0.000
SummarizedExperiment::assays(rse) <- S4Vectors::SimpleList(counts=imputed_counts)
SummarizedExperiment::assay(rse)
#> A B C D
#> [1,] 2876.4876 8895.5036 1428.8574 6651.486831
#> [2,] 7883.2630 6928.3413 4146.0488 949.311769
#> [3,] 4090.3602 6405.4276 4137.8295 3840.312408
#> [4,] 8830.2910 9942.7035 3689.0857 2744.562062
#> [5,] 9404.7324 6557.4023 1525.2950 8146.585749
#> [6,] 4439.2947 7085.5962 1388.9218 4485.714898
#> [7,] 5281.5268 5441.1162 2331.1080 8100.833466
#> [8,] 8924.2980 5941.8261 4660.1585 8124.082706
#> [9,] 5514.7987 2892.3082 2660.4604 7943.628869
#> [10,] 4566.6907 1471.9894 4812.3872 4398.877044
#> [11,] 9568.3766 5545.7565 459.2658 7544.997111
#> [12,] 4533.8882 9023.0882 4422.5585 4611.837102
#> [13,] 6776.0288 6907.3621 7989.4495 7102.113831
#> [14,] 5726.7614 7954.8787 1219.8707 7.247108
#> [15,] 1030.1439 247.1122 5609.9189 4753.690424
#> [16,] 8998.3499 4778.4819 2066.1074 2201.968733
#> [17,] 2461.6313 7584.8369 1276.1890 3798.785561
#> [18,] 421.5533 2164.8630 7533.3253 6128.097262
#> [19,] 3279.8793 4566.2289 8950.5585 3518.627295
#> [20,] 9545.0820 2317.0262 3745.2533 1112.243108
SummarizedExperiment::assays(rse) <- S4Vectors::endoapply(SummarizedExperiment::assays(rse), asinh)
SummarizedExperiment::assay(rse)
#> A B C D
#> [1,] 8.657472 9.786448 7.957778 9.495743
#> [2,] 9.665644 9.536523 9.023058 7.548885
#> [3,] 9.009536 9.458048 9.021074 8.946456
#> [4,] 9.779090 9.897741 8.906281 8.610524
#> [5,] 9.842115 9.481497 8.023090 9.698501
#> [6,] 9.091398 9.558966 7.929430 9.101800
#> [7,] 9.265118 9.294887 8.447246 9.692869
#> [8,] 9.789680 9.382919 9.139952 9.695735
#> [9,] 9.308338 8.662957 8.579402 9.673273
#> [10,] 9.119691 7.987517 9.172096 9.082252
#> [11,] 9.859366 9.313936 6.822778 9.621787
#> [12,] 9.112482 9.800689 9.087621 9.129529
#> [13,] 9.514294 9.533490 9.679024 9.561295
#> [14,] 9.346053 9.674688 7.799647 2.678476
#> [15,] 7.630601 6.202994 9.325439 9.159824
#> [16,] 9.797944 9.165025 8.326569 8.390254
#> [17,] 8.501727 9.627054 7.844781 8.935584
#> [18,] 6.737095 8.373260 9.620239 9.413787
#> [19,] 8.788709 9.119590 9.792618 8.858973
#> [20,] 9.856929 8.441187 8.921392 7.707281
SummarizedExperiment::rowRanges(rse)
#> GRanges object with 20 ranges and 1 metadata column:
#> seqnames ranges strand | feature_id
#> <Rle> <IRanges> <Rle> | <character>
#> [1] chr1 409164-409167 - | ID001
#> [2] chr1 691082-691085 + | ID002
#> [3] chr1 388335-388338 + | ID003
#> [4] chr1 268922-268925 - | ID004
#> [5] chr1 804064-804067 - | ID005
#> ... ... ... ... . ...
#> [16] chr2 647861-647864 + | ID016
#> [17] chr2 469620-469623 + | ID017
#> [18] chr2 232385-232388 + | ID018
#> [19] chr2 941769-941772 - | ID019
#> [20] chr2 371106-371109 + | ID020
#> -------
#> seqinfo: 2 sequences from an unspecified genome; no seqlengths
SummarizedExperiment::rowData(rse)
#> DataFrame with 20 rows and 1 column
#> feature_id
#> <character>
#> 1 ID001
#> 2 ID002
#> 3 ID003
#> 4 ID004
#> 5 ID005
#> ... ...
#> 16 ID016
#> 17 ID017
#> 18 ID018
#> 19 ID019
#> 20 ID020
SummarizedExperiment::colData(rse)
#> DataFrame with 4 rows and 1 column
#> Treatment
#> <character>
#> A ChIP
#> B Input
#> C ChIP
#> D Input